Artificial Intelligence (AI) has rapidly advanced in recent years, transforming various aspects of our lives, from healthcare to finance, and from education to entertainment. While AI offers numerous benefits, it also brings with it the potential for bias and discrimination. In this blog post, we will delve into the issues of bias and fairness in machine learning, exploring their causes, consequences, and the strategies to address them.
Understanding Bias in Machine Learning
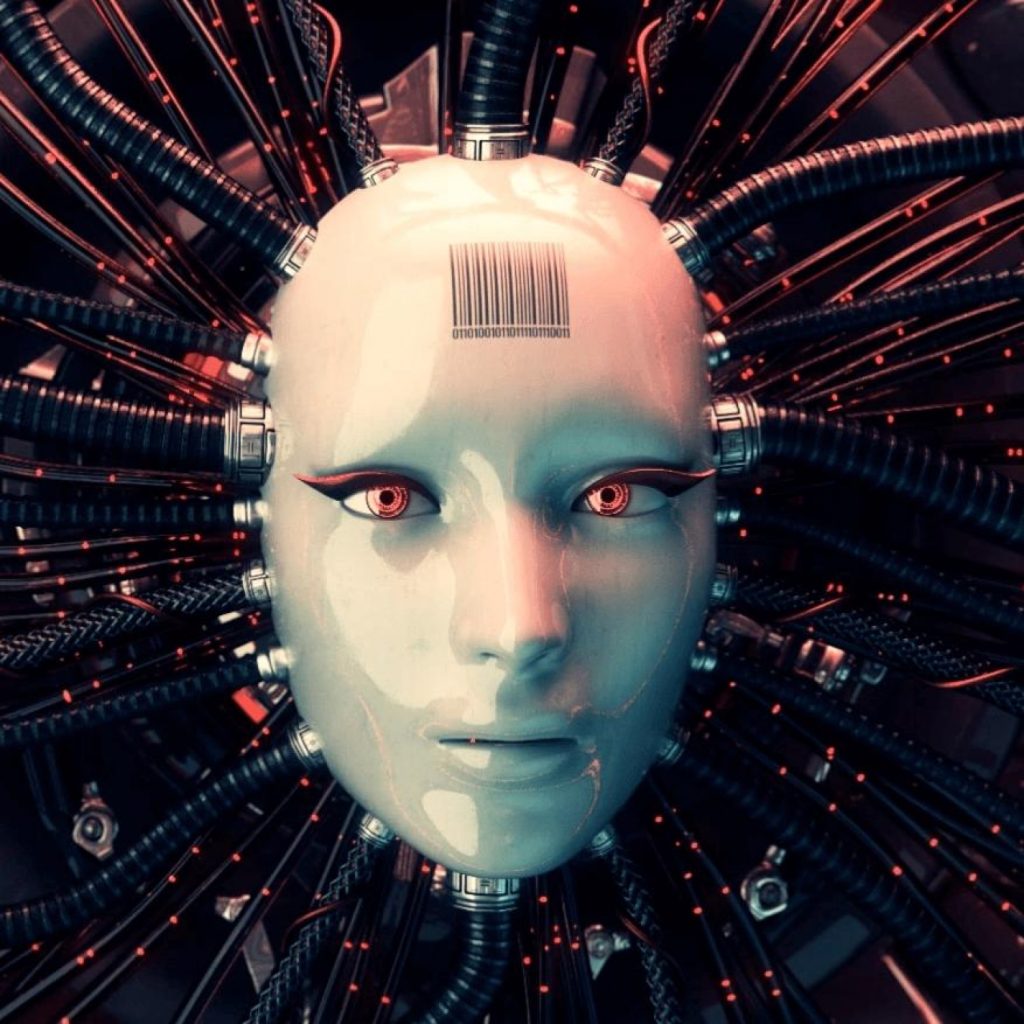
Bias in AI systems arises when the data used to train these systems is not representative of the real-world population they are meant to serve. This bias can manifest in various ways, including racial, gender, or socioeconomic disparities. For example, if an AI system is trained on historical data that reflects biased human decisions, it can perpetuate and even exacerbate those biases when making predictions or decisions.
The Consequences of Bias in AI
The consequences of bias in AI are far-reaching and can have a detrimental impact on individuals and communities. Biased algorithms can result in unfair treatment in various domains. For instance, biased facial recognition systems may misidentify or under-identify certain racial or ethnic groups, leading to unjust surveillance or access denial. Biased lending algorithms can discriminate against marginalized communities, making it harder for them to access loans and financial resources.
The Role of Fairness in Machine Learning
To address bias in AI, fairness becomes a critical consideration. Fairness in machine learning refers to the objective of ensuring that AI systems treat all individuals or groups fairly and without discrimination. Achieving fairness is a complex task, as it requires both technical and ethical considerations.
Causes of Bias in AI
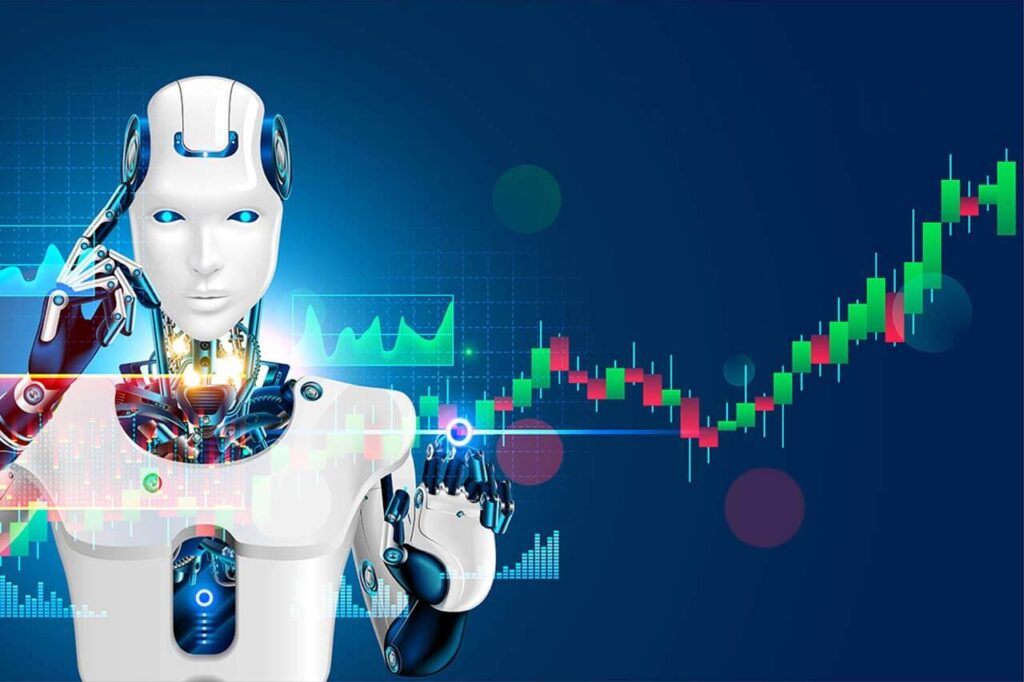
Bias in AI can be introduced at multiple stages of the machine learning pipeline. One common source of bias is biased data collection. If the data used to train a model is not diverse or representative, the model will inevitably inherit those biases. Additionally, bias can be introduced during the preprocessing of data or through the algorithmic design itself.
Strategies to Address Bias and Enhance Fairness
Addressing bias in AI is a multifaceted challenge that requires a combination of technical and ethical solutions. Here are some strategies that can help mitigate bias and enhance fairness in machine learning:
1. Diverse and Representative Data
To combat bias, it’s crucial to ensure that the training data is diverse and representative of the population the AI system will serve. This may involve collecting more comprehensive and inclusive datasets and actively seeking to address underrepresented groups.
2. Bias Detection and Mitigation
Developing tools and techniques for detecting and mitigating bias in AI models is essential. This can involve incorporating fairness metrics into the model evaluation process and implementing algorithms that reduce bias in predictions.
3. Transparency and Accountability
Promoting transparency in AI development and deployment is vital. Organizations should document their data sources, algorithms, and decision-making processes, making them accessible for scrutiny and auditing. This helps hold developers and systems accountable for any bias or discrimination that may occur.
4. Ethical Guidelines and Regulations
Governments and industry organizations should establish clear ethical guidelines and regulations for AI development and use. These guidelines can help set standards for fairness, transparency, and accountability in AI systems.
5. Continuous Monitoring and Improvement
Addressing bias and fairness in AI is an ongoing process. Organizations should continuously monitor their AI systems and update them as necessary to ensure they remain fair and free from bias. In the age of AI, addressing bias and promoting fairness in machine learning is a pressing challenge. While there is no one-size-fits-all solution, a combination of technical advancements and ethical considerations can help mitigate bias and ensure that AI systems are fair and just in their interactions with individuals and communities. As AI continues to play an increasingly significant role in our lives, it is imperative that we prioritize fairness and equity in its development and deployment.